Gianluca Demartini, Ph.D.
School of Information Technology and Electrical Engineering,
University of Queensland
St Lucia
QLD 4072 Australia
Office: +61 7 336 58325
demartini@acm.org
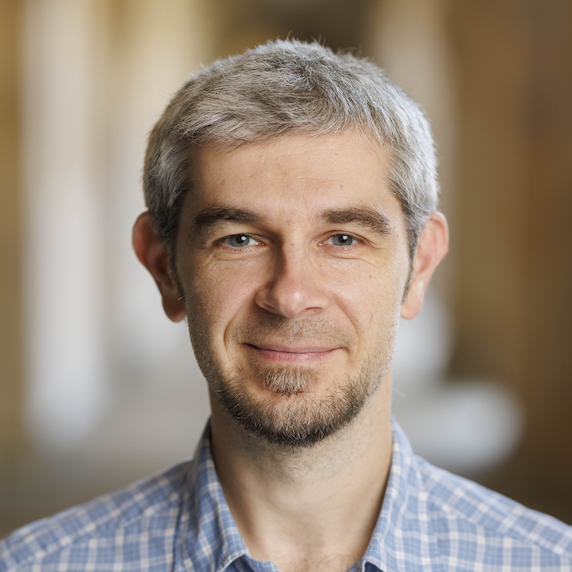
Dr. Gianluca Demartini is an Associate Professor in Data Science at the University of Queensland, School of Electrical Engineering and Computer Science. His main research interests are Information Retrieval, Semantic Web, and Human Computation. His research has been supported by the Australian Research Council (ARC), the Swiss National Science Foundation (SNSF), the EU H2020 framework program, the UK Engineering and Physical Sciences Research Council (EPSRC), Facebook, Google, and the Wikimedia Foundation. He received Best Paper Awards at the ACM SIGIR International Conference on the Theory of Information Retrieval (ICTIR) in 2023, AAAI Conference on Human Computation and Crowdsourcing (HCOMP) in 2018 and at the European Conference on Information Retrieval (ECIR) in 2016, the Best Short Paper Award at ECIR in 2020 and the Best Demo Award at the International Semantic Web Conference (ISWC) in 2011. He has published more than 200 peer-reviewed scientific publications including papers at major venues such as WWW, ACM SIGIR, VLDBJ, ISWC, and ACM CHI. He has given several invited talks, tutorials, and keynotes at a number of academic conferences (e.g., ISWC, ICWSM, WebScience, and the RuSSIR Summer School), companies (e.g., Facebook), and Dagstuhl seminars. He is a senior member of the ACM since 2020, an ACM Distinguished Speaker since 2015, and has been a TEDx speaker in 2019. He serves as associate editor for the Transactions on Graph Data and Knowledge (TGDK) Journal and as an editorial board member for the Information Retrieval journal. He is a steering committee member for the AAAI HCOMP conference. He was PC Chair for the ACM Conference on Research and Development in Information Retrieval (SIGIR) in 2022. He was General co-Chair for the ACM International Conference on Information and Knowledge Management (CIKM) 2021. He was Crowdsourcing and Human Computation Track co-Chair at WWW 2018 and co-chair for the Human Computation and Crowdsourcing Track at ESWC 2015. He has been Senior Program Committee member for, among others, the ACM Conference on Research and Development in Information Retrieval (SIGIR), the ACM Web Search and Data Mining (WSDM) Conference, the International Joint Conference on Artificial Intelligence (IJCAI), the AAAI Conference on Human Computation and Crowdsourcing (HCOMP), and the International Conference on Web Engineering (ICWE). He co-organized several workshops and tutorials at international conferences as well as the Entity Ranking Track at the Initiative for the Evaluation of XML Retrieval in 2008 and 2009. Before joining the University of Queensland, he was Lecturer at the University of Sheffield in UK, post-doctoral researcher at the eXascale Infolab at the University of Fribourg in Switzerland, visiting researcher at UC Berkeley, junior researcher at the L3S Research Center in Germany, and intern at Yahoo! Research in Spain. In 2011, he obtained a Ph.D. in Computer Science at the Leibniz University of Hanover focusing on Semantic Search.
Algoritmo di Landau-Vishkin: implementazione
Tesi in formato pdf (scaricata 3699 volte da luglio 2011)
Un esempio in cui è appropriato l’uso di limitare il numero di differenze nell’allineamento di due sequenze biologiche è quello della localizzazione di geni le cui mutazioni causano, o contribuiscono, a certe malattie genetiche. Vengono confrontate sequenze di DNA che rappresentano geni di persone malate e di persone sane per trovare le differenze consistenti dato che molte malattie genetiche sono causate da cambiamenti molto piccoli in un gene.
Per risolvere questi problemi sono stati prodotti diversi algoritmi prima di quello proposto da Landau e da Vishkin, come ad esempio quelli basati su tecniche di programmazione dinamica o
quello proposto da E. Ukonnen con complessità comunque peggiori di quello qui studiato. Si vuole implementare l’algoritmo descritto da Gad M. Landau e Uzi Vishkin nell’articolo intitolato “Fast String Matching with k differences” e pubblicato nel 1988 sul “Journal of Computer and System Sciences”. L’obiettivo di questo algoritmo è di scoprire tutte le occorrenze di una stringa P
in un testo T accettando al più k errori durante il confronto.
In questo documento verrà dapprima presentato il problema generale dell’allineamento tra stringhe con le necessarie definizioni dei concetti essenziali, e quindi verranno presentati degli algoritmi che sono poi stati migliorati nel tempo fino a descrivere in dettaglio l’algoritmo di Landau-Vishkin presentando quali sono i suoi vantaggi rispetto ai precedenti. In fine vi sarà una descrizione del codice prodotto per realizzare l’implementazione dell’algoritmo studiato.
Per produrre l’implementazione dell’algoritmo di Landau-Vishkin si è per prima cosa implementato un algoritmo più semplice che risolve lo stesso problema con complessità peggiore (quello di Ukonnen), per poi modificare la parte fondamentale mantenendo la struttura del precedente, come fatto da Landau e da Vishkin con le loro idee. Un vantaggio dell’implementazione proposta è che i risultati vengono inseriti in un file e quindi possono essere riutilizzabili successivamente da altre applicazioni. Il difetto principale dell’implementazione invece è la sua complessità quadratica. Oltre al necessario miglioramento della complessità sarebbe utile sostituire l’utilizzo di file con l’utilizzo 6 di database sia per contenere le stringhe di input sia per contenere i risultati in output, in quanto la dimensione di questi, nell’utilizzo che ci si propone di effettuare, si suppone sia molto elevata e quindi si presenterebbero dei problemi di memoria fisica insufficiente a contenere i dati.
1. Introduzione
2. Allineamento di sequenze con errori
3.
Contesto storico
4. Un algoritmo inefficiente per
l’allineamento di stringhe con errori
5. Algoritmo di
Landau-Vishkin
5.1 Struttura dell’algoritmo
5.2
Suffix Tree
5.2.1 Trovare il Lowest-Common-Ancestor in tempo
costante
5.3 Pseudo-codice dell’algoritmo di
Landau-Vishkin
5.4 Complessità dell’algoritmo di
Landau-Vishkin
5.4.1 Complessità utilizzando un suffix
tre
6. Descrizione del codice sorgente
6.1 File Alg.H
6.2 File Main.C
6.2.1 La funzione main
6.3 File Triple.C
6.3.1 La funzione addTriple
6.3.2 La funzione findCover
6.4 File BuildMaxLen.C
6.4.1 La funzione build_maxlen
6.5
File File.C
6.5.1 La funzione openpattern
6.5.2 La
funzione opentext
6.5.3 La funzione writeresults
6.5.4 La
funzione fsize
6.6 File Check.C
6.6.1 La funzione check
7. Testing dell’implementazione
8. Conclusioni
Bibliografia